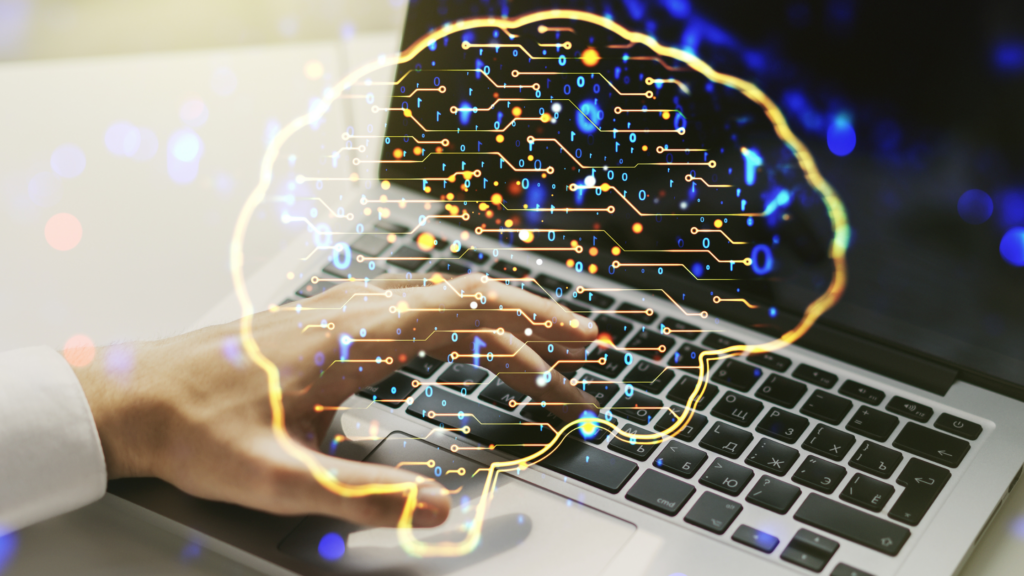
In the dynamic realm of machine learning, the power to enhance predictive accuracy and unlock new insights lies in the utilization of external data sources. While internal data is valuable, integrating external data can provide a broader perspective and contribute to more robust models. In this blog, we’ll explore four compelling methods to harness external data for enhancing your machine learning models.
1.Enriching Feature Space with External Data:
The richness of your feature space directly impacts the quality of your model. By incorporating relevant external data, you can introduce new dimensions to your feature space that might not have been available in your initial dataset. For instance, if you’re building a recommendation system for an e-commerce platform, including data from social media platforms about users’ interests and interactions can significantly enhance your model’s ability to make accurate suggestions. However, it’s important to ensure that the external data aligns with your problem statement and is of high quality.
2. Transfer Learning for Improved Generalization:
Transfer learning is a powerful technique that leverages knowledge learned from one task to enhance the performance of another. When it comes to machine learning models, this translates into using pre-trained models on large datasets and fine-tuning them for your specific task. This approach is particularly useful when external data is scarce or expensive to collect. For example, in image classification, you can take a pre-trained convolutional neural network (CNN) and fine-tune it on your dataset, allowing the model to learn features from both your data and the external data on which it was initially trained.
3. Data Augmentation for Improved Robustness:
Data augmentation involves artificially increasing your dataset’s size by applying various transformations to your existing data, such as rotating, cropping, or flipping images. While this might not be traditional “external data,” the concept is similar in that it introduces variability from outside the original dataset. Incorporating augmented data can help improve your model’s ability to generalize and perform well on new, unseen data. For instance, in natural language processing, you can generate paraphrased versions of your text data to expose the model to different sentence structures.
4. External Data for Contextual Insights:
External data sources can provide contextual information that enriches your model’s understanding of the world. For example, if you’re building a weather prediction model, incorporating geographical and atmospheric data from external sources can help your model better comprehend the broader environmental factors that contribute to weather patterns. This contextual understanding can lead to more accurate predictions and a deeper grasp of the underlying relationships.
Enhancing Your Machine Learning
In conclusion, the integration of external data into your machine learning models can be a game-changer. From enriching your feature space to enhancing generalization and robustness, external data sources offer a wealth of possibilities. However, it’s crucial to approach this process thoughtfully. Ensuring the quality, relevance, and compatibility of external data with your problem is paramount. When executed effectively, these strategies can elevate your models to new levels of accuracy and insight.
Remember, the art of using external data lies not just in its incorporation, but in the intelligent fusion of domain knowledge, data preprocessing, and model design. As the machine learning field continues to evolve, the ability to harness the potential of external data will remain a vital skill for creating cutting-edge models.